The Evolution of AI: Where It Started and Where It’s Headed
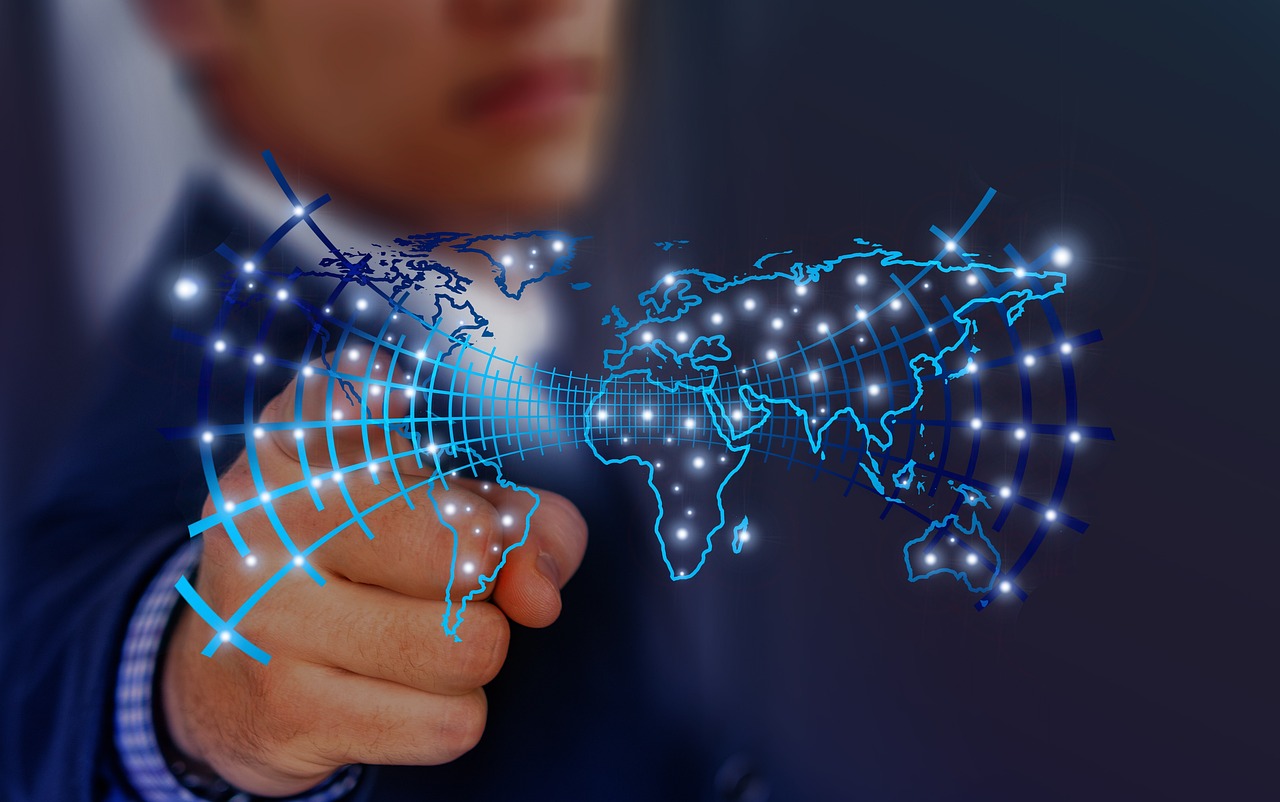
The evolution of artificial intelligence has been marked by periods of rapid progress followed by extended winters of reduced funding and interest. However, in recent years AI has made dramatic leaps forward in capabilities fueled by new algorithms, vast data sets, and increased compute power. As mainstream adoption continues accelerating, examining the winding trajectory of AI can provide perspective on how we arrived at the current state and where the technology may head next.
This essay traces the history of highs and lows in AI advancement while analyzing forces shaping its future. It covers early optimism and setbacks in AI research, breakthroughs finally enabling machine learning takeoff, expansions to the edge of automation, as well as societal implications and looming regulation that could dictate the pace moving forward. The essay seeks to demonstrate how despite volatility in progress, AI will indelibly continue enhancing decision making while gradually blending deeper into daily life when guided responsibly.
The Origins of AI
Before chronicling the evolution of capabilities, it is important to distinguish artificial intelligence itself. At its core, AI refers to computational systems designed to perform tasks we associate with human intelligence like visual perception, speech recognition, and decision making. The spectrum of intelligence ranges from niche or “narrow” AI able to exceed human capability on a singular task all the way to “general” artificial intelligence rivaling the full span of human cognition. While general AI does not yet exist, extraordinary progress has been made across more narrowly focused applications.
While recent successes have been swift and striking, AI research originated decades ago. In the 1950’s scientists began exploring machine learning algorithms that could “learn” from data to complete tasks like playing checkers as opposed to being explicitly programmed. This spawned optimism that human level intelligence would soon be replicated. However, researchers underestimated the complexity of common sense reasoning. By the 1970’s funding declined dramatically leading into the first of several “AI winters” where skepticism doused development. It would take later converging breakthroughs to spur the period of exponential advancement we see today.
Advancements in Machine + Deep Learning
The resurgence and rapid acceleration of AI over the past 15 years traces back to revelations in machine learning and neural networks. Machine learning refers to programmatic techniques where algorithms independently model data to uncover patterns and make predictions without scripted rules. Neural networks expand upon this through layered architectures loosely modeled after the human brain to interpret rich stimuli like images as input.
In the 1990’s and 2000’s machine learning gained traction through Support Vector Machines classifying spam email and Hidden Markov Models enabling speech interfaces. However, the disruptive breakthrough emerged in 2012 when professor Geoffrey Hinton’s team won the ImageNet competition using a deep convolutional neural network architecture to classify images with far greater accuracy than prior approaches. Computer vision had long struggled with the nuance of perceiving pixel values rather than rule-based features. Deep learning offered a path by processing images through cascading neural network layers each detecting higher level visual features. This discovery around deep learning for perceptual tasks sparked the modern wave of AI investment and mania around its potential.
Capital, Data and Compute Power
The imagenet moment served as ignition, but several other key factors have fueled rapid progress in AI since its win. First, funding flooded back into the space after the deep learning breakthrough. AI startups raised $70 billion between 2015 and 2021 as corporations and investors rushed to harness its possibilities after years of indifference. This influx financed talent expansion and innovation.
Secondly, new large annotated data sets created the raw material needed to train advanced models. ImageNet itself contained 14 million categorized images. New repositories provided similar mass data for video, speech, and language. Previously AI showed promise but lacked robust training data to maximize potential.
Finally, compute power dramatically increased through affordable parallel graphics processing units (GPUs) that excelled at neural network matrix math. Combined with custom silicon chips from startups like Cerebras designed for model efficiency, AI workloads previously requiring supercomputers can now leverage cost-efficient infrastructure available through cloud platforms.
The combination of renewed excitement, capital, data and compute collectively put decades of foundational research into fruitful motion highlighting AI’s latent potential while yielding adaptive flexible systems less reliant on rigid programming.
Current State of AI
Years of compounding algorithmic and architectural innovations have yielded AI systems with incredible capacity across applications. Key areas demonstrating surging intelligence include:
Computer Vision
From satellite image classification to video surveillance monitoring and medical scan analysis, computer vision now rivals and often exceeds human capability.
Conversational AI
Smart assistants like Alexa, auto-complete email responses, and customer service chatbots display expanding language fluency and contextual dialogue abilities.
Recommendation Engines
Platforms like Netflix and Spotify learn individual interests to predict content someone will enjoy with continually improving accuracy.
Autonomous Vehicles
Cars able to independently navigate environments show progress with Waymo driverless taxis surpassing 20 million miles driven in 2020.
Fraud + Threat Detection
AI helps flag network intrusions, fraudulent transactions, spam accounts, and even potentially dangerous extremist content through pattern identification.
As these examples of narrow applications indicate, AI flexibility to ingest training data for specialized learning has proven valuable across domains though truly general capability remains lacking.
Current Barriers to Further Progress
While practical AI has started automating an increasing number of tasks, current systems still face notable limitations holding broader progress back including:
Brittleness
ML models remain fragile and unreliable when faced with novel inputs that deviate far from training data distributions. Unforeseen edge cases easily confuse otherwise highly accurate AI.
Explainability
The statistical correlations AI learns do not provide human understandable explanations behind predictions made, keeping decision logic a “black box” ill-suited for many business settings.
Data Constraints
Certain real-world domains like healthcare have far less reliably labeled training data available slowing application development despite massive potential value.
Energy Consumption
Large modern neural networks require substantial computational resources to run contributing growing carbon footprints. More efficient architectures are needed.
Trust + Acceptance
Limited transparency into AI decision making processes hampers full confidence and adoption among wary individuals and corporations despite proven capabilities.
While expanding datasets, advanced chipsets and new structural techniques aim to chip away at these limits, they remain obstacles to realizing more expansive AI deployment.
The Future Trajectory of AI
Though AI has come far in replicating facets of intelligence, the road ahead likely holds much greater potential as more foundational barriers to advancement are overcome. Key directions predicted in coming years include:
Continued Specialization
While hype exists around artificial general intelligence, pragmatic focus will remain on specialized neural networks exceeding humans in targeted applications versus flexible general systems.
Embedded Decisions
Rather than AI operating as an add-on, predictive intelligence will embed closer to data and systems enabling automated responsive adaptations without human involvement.
Offline Functionality
On-device inference will allow AI processing even with limited connectivity rather than relying solely on cloud platforms to enable localization and privacy.
Generative Applications
Models for creating original content like images, video, and text rather than just classification/prediction will enrich products and scale creative workflows.
Democratized Development
Automated machine learning (AutoML) will enable those lacking specialized skills to train models through intuitive interfaces and proactive assistance further expanding adoption.
As this trajectory highlights, AI will shift behind the scenes enhancing ambient user experiences through responsive anticipation of needs while aiding business decisions and creative endeavors. Smarter embedded intelligence with wider accessibility stands to compound progress.
Broader Implications for Society
The evolution of AI will shape societal shifts as much as technical ones. As automation increases, fundamental questions around impacts to jobs, privacy, bias and regulation arise:
Workforce Disruptions
Tasks currently performed by humans even experts face rising threat of replacement by AI systems exceeding human capability with lower costs at higher scales. Transition plans are needed at a policy level to account for substantial labor market disruptions.
Data Vulnerability
Vast data required for accurate AI risks exposure, while behavioral data collection required for personalization raises privacy concerns despite utility improvements. Ethics around data rights and transparency are needed.
Accountability Gaps
Harm caused by model errors or encoded biases around gender, race and ethnicity require traced back to responsible parties, yet current vendor ecosystems pose attribution challenges clouding accountability.
Regulatory Catch-up
Governance frameworks struggle to keep pace with technology progress as capabilities outgrow current regulations. Collaborative regulation that protects interests yet promotes innovation will pose ongoing challenges.
While technical evolution yields boundless positive potential, it simultaneously strains social constructs surrounding jobs, privacy, bias, and control. Progress must pair with philosophical evolution centered on human dignity, fairness and shared prosperity to maximize benefit.
Looking Ahead
With innovators rapidly addressing barriers every year, AI stands poised to build upon progress made in replicating visual, vocal and logical human intelligence across domains. Each new breakthrough expands what is within reach – affirming AI’s permanence while raising important questions about responsible collaboration between its algorithmic capabilities and human values. While forecasts remain uncertain, identifying current limitations illuminates that in many ways artificial intelligence remains in its infancy with a promising if precarious journey ahead. Its future contours will depend profoundly on continual engagement between technology, policy, and society to ensure innovations elevate rather than erode human potential and plurality.
Conclusion
The evolution of AI has been marked by punctuated eras of enthusiasm and subsequent disillusion tied to cycles of overpromising followed by technical bottlenecks. Today’s surge results from a combustible mixture of algorithmic insights, data abundance and affordable parallel compute power that finally unlocked lengthy theoretical research. Recent exponential gains in practical applications have yielded an ever-expanding repertoire of intelligent assistance now spanning industries and permeating daily life. But as adoption continues accelerating, AI again faces skeptical scrutiny around limitations and implications requiring nuanced navigation and governance. If steered responsibly, AI systems enhance rather than endanger human potential – helping illuminate knowledge, automate tedious tasks, quicken discoveries and restore time for more meaningful pursuits. The past leaves little doubt this symbiotic relationship will continue cementing artificial intelligence as an enduring ally to human progress when guided collectively by empathy.